ChatGPT for Ad Tech: Steps to Build an AI Strategy for Brands
by Mathew Broughton on 31st Jan 2023 in News
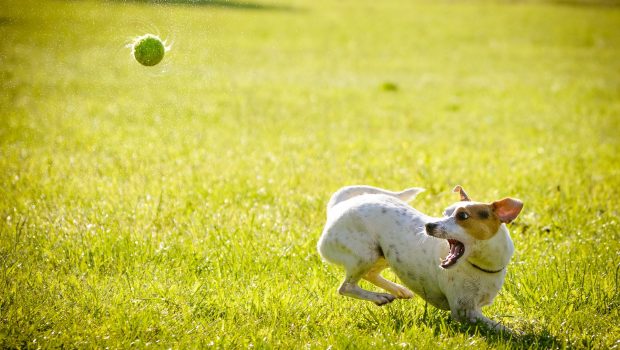
In association with Scibids.
The emergence of ChatGPT has been a mainstay of social feeds and discussions across the marketing ecosystem in recent weeks. In light of this and other new generative AI platforms, Remi Lemonnier, CEO, Scibids, discusses the implications for the ad tech industry, and how brands can capitalise upon these solutions.
It would be an understatement to say that AI is the new “it” term. Thanks to the hype around ChatGPT, generative AI is all the rage for consumers, technicians, and venture capitalists alike. But what does the technology mean for the ad tech ecosystem? Well, while the uses of AI may be new to some industries, it certainly isn’t new for ad tech.
When Scibids was founded in 2016, its AI technology was built to raise expectations in the performance of digital marketing. For me, AI continues to mean creating purpose-built solutions that respect privacy and consumers, while increasing automation to ease operational complexity and open up scale potential.
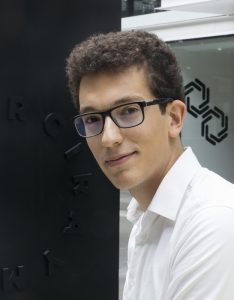
There are few industries like digital marketing which present such an ideal environment for deploying AI and machine learning. The scale of the sector is global and growing, the operational challenges are mighty, and data availability is near real time. Not to mention that few industries are as important as advertising, which enables our global marketplace of ideas to thrive. For example, a 2022 study by The Advertising Coalition found that “advertising generated USD$7.1tn (£5.7tn) in sales activity" in the US alone in 2021.
According to the Macroeconomic Impact of Artificial Intelligence report by PwC,
AI will be the number one driver of GDP growth by 2030. We’ve quoted this stat for a while now but still marvel at how AI has impacted so many different industries worldwide. So, what does the development of generative AI and ChatGPT actually mean for digital marketing and ad tech?
First, let’s understand what AI actually is. One thing to keep in mind, which I learned when attending leading AI conferences during my Ph.D. days, is the extreme diversity of topics that fall under AI’s umbrella. From modeling news diffusions in social networks to self-driving cars.
As a true AI enthusiast, I decided to ask the question, 'What is the definition of artificial intelligence?', to ChatGPT itself, which provided the following answer:
"Artificial intelligence (AI) refers to the simulation of human intelligence in machines that are programmed to think and learn like humans. This includes the ability to understand natural language, solve problems, make decisions, and adapt to changing situations. AI systems can be trained using various techniques such as machine learning, deep learning, and natural language processing to perform tasks that typically require human intelligence."
This definition is not wrong, but I must beg to differ. Instead, I tapped into RemiGPT (that would be my brain), which disagreed with the portion of the response that states, “programmed to think and learn like humans.” It’s a common misconception to believe that AI can think and learn like humans. However, by design, AI can’t actually do that. The human brain is incapable of processing the gigantic amounts of data that AI can. AI is therefore able to come up with new approaches and analyses that a human could not possibly do!
Moreover, the definition gives the impression that a general AI exists, which is not the case. It altogether lacks acknowledgment of the evolution of automation from the repetitive tasks of factory workers to tasks nessecitating high cognitive efforts.
Instead, I prefer this definition of AI: Automation of non-trivial tasks that traditionally required human intelligence based on a learning system fed with data.
With this definition of AI established, let’s now dive into the difference between generative AI versus predictive AI.
Generative AI: generates new content or data based on patterns and information it has learned from previous data. A generative AI model can be trained to generate new text, images, or music. But there is no actual benchmark for performance.
Predictive AI: predicts future outcomes using past data. A predictive AI model can predict stock prices, customer behavior, and conversion probabilities in digital advertising, with success being measurable and provable.
Now, let’s consider trends in these two technologies for the years to come…
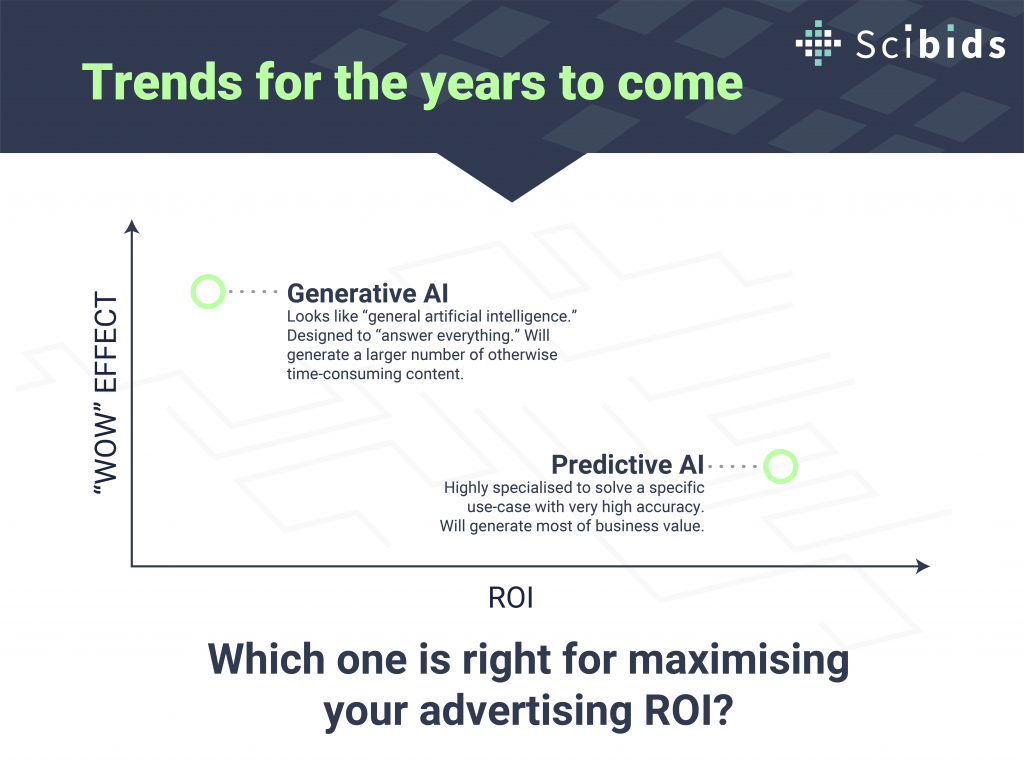
So, which of the trends is suitable for maximising advertising ROI? After working with dozens of sophisticated brands to strengthen their intelligence layer for digital marketing, purpose-built AI over generative AI is desired by the market and produces the most business value. Now, let's dive deeper into what we see as three key components of a mature digital brand's tech stack and see how they are related to AI: measurement, brand safety and bidding.
Measurement is necessary for brands to define what they are trying to achieve and measure ROI. This is mostly rules-based logic, meaning that it will consistently produce the same output for a given input.
Meanwhile, brand safety, necessary to protect brands against harmful content or fraud, is a blend between rules-based and logic and AI methods. It taps into natural language processing (NLP) and neural networks. These are categories of AI that focus on building systems that can learn from and make decisions based on data. Brands can also leverage computer vision AI to detect harmful video content for brand safety purposes.
Lastly, bidding (therefore, ad decisioning) represents the billions of decisions brands must make daily in the media buying process. It is the most critical AI-based application for digital marketing because it has the most impactful use and it is precisely where other components like brand safety and measurement can be valued and activated. It will define a brand’s performance by finding the probability of the desired outcome. Brands need to control optimisation and the intelligence they bring to the table to fuel it to create their own competitive advantage and ROI while ensuring the most efficient price at scale.
Bidding is 100% AI-based when customisable algorithms are used. When I started in digital marketing over a decade ago, bids were sometimes manually placed on strategies. Essentially, human traders guessing what a bid maximum should be! However, this doesn’t make sense as each impression opportunity is valued differently and should be priced separately.
Brands are increasingly asking for more transparency in their media buys and understanding the data and objectives behind ad decisioning. They are also progressively asking to optimise towards quality CPMs (Q-CPMs), quality metrics, and business goals, that are more than just clicks and standard KPIs.
AI needs to be trained to deliver performance without using PII for targeting to respect consumers and ensure spend is optimised 100% towards maximising ROAS. Bidding AI needs to be purpose-built, customisable, and dynamic, add learnings from past campaigns to future campaigns, and consistently create new opportunities for automation to improve campaign scale.
Customisable algorithms are therefore the AI technology, data, and people training them. All three components must be sophisticated to compute and produce the outcomes brands want. Think of it in these simple terms: You can have the most intelligent dog out there, but if you can’t throw the ball in the right direction, your dog will never learn where to go!
Ad FraudAIBiddingBrand SafetyBrand SuitabilityMeasurement
Follow ExchangeWire