Why Marketers Need to Be More NASA
by Lindsay Rowntree on 4th Mar 2016 in News
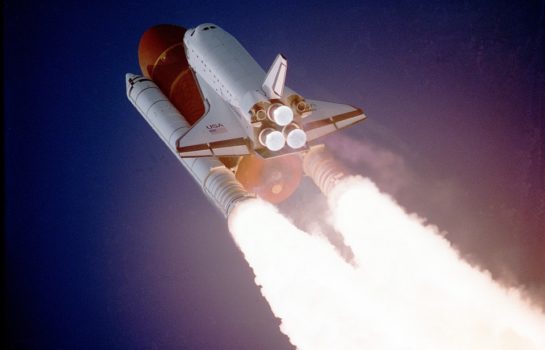
As data volumes have increased exponentially in recent years, the ability to sift through and identify the important insights has become an increasingly sought-after skillset. Industries as diverse as Finance, Professional Sports, and Healthcare are now recruiting people with data handling and analysis expertise to help steer strategic decision making. But what kind of value can these people bring to the marketing world? Jenny Thompson (pictured below), head of data science & advanced analytics, Forward3D, speaks to ExchangeWire about the three ways a data scientist can supercharge your performance marketing, and how NASA may be a good example to follow.
How to build a rocket
One of NASA’s fundamental goals has always been to gain a better understanding of the universe, with the intention of using this knowledge for the betterment of all of us on Earth. In order to achieve this goal, they had to achieve the unthinkable – sending human beings into space a mere 58 years after the first flight in a powered airplane.
To build a rocket capable of doing this, they identified a long list of technical challenges related (but not limited) to:
– Propulsion
– Trajectory
– Tensile Strengths
– Electromagnetics
In order to solve the problems posed, NASA was going to need a new breed of scientists who had expertise across a range of skills including Chemistry, Maths, Physics, Mechanical Engineering and Computer Science. NASA needed rocket scientists.
Marketing propulsion systems
Brands also (metaphorically) occupy their own distinct universe, abundant with data resources that can furnish great benefits, if handled and analysed correctly. In order to make sense of this unique space and deliver effective, evidence-based campaigns, marketers also face a set of distinct challenges that stand in their way, relating to data silos, data volume, data variety, data velocity, and data veracity.
Just as NASA needed to bring together a set of complementary skills, so do marketers. Maths and statistics expertise, marketing domain knowledge, and creative programming acumen are all required to meet the challenges above.
Bringing multiple skill sets together into a new approach has, again, introduced a requirement for a distinct profession. Brands need data scientists.
How data scientists can help
There are three quick ways in which a data science team can apply this mix of skills to help clients understand their universe and increase the effectiveness of their performance marketing.
Dynamic attribution modelling:
Technical challenges overcome: data silos, data volumes, data variety
A huge challenge for marketers trying to understand their brand universe is gaining an accurate understanding of how customers discover, engage with, and are influenced by their marketing communications and media touch points. Many attribution models will place too great an emphasis on last-click channels, ignoring or under representing channels that may have played a crucial role earlier in the purchase process.
This challenge can be overcome by developing a dynamic model that allocates weights algorithmically. By breaking down data silos to focus on the individual customer path, the model can calculate weightings based on performance and channel synergies observed across all tracked customer paths. Weights adjust to account for seasonality, overall trend and campaign effectiveness on a daily basis, requiring an expertise on the data-science end in handling immense data volumes.
Customisations to incorporate specific profitability calculations, and custom segmentation for brands, means that the outputs are tailored to help them solve challenges such as identifying the channels that are most effective at driving new customer acquisition in a specific market. Data is merged from a variety of sources and structures, such as web tracking, customer CRM systems, and pricing systems, in order to achieve this aim.
With a focus on customer paths, more meaningful metrics and customisations based on specific requirements, strong attribution modelling systems give brands the power to make better investment and more effective optimisation decisions.
Profit erosion modelling:
Technical challenges overcome: data silos, data volume
Reporting on marketing activities often only looks at gross revenue. This can be a misleading and, in some cases, a harmful metric to use for decision-making purposes as it doesn’t show the actual benefit to the business. A product may drive a huge amount of revenue, for instance, but also have high costs associated with shipping, fulfillment, product margin, or advertising. This could mean this item is actually less profitable than another product that drives less overall revenue, but has lower associated costs. It is crucial for businesses to understand which elements are eroding their overall profit so that they can make adjustments to advertising, pricing, or merchandising activities. In order to overcome this challenge, data scientists can break down data silos across brands in order to obtain and create a rich dataset. They can then run analysis at scale in order to pull valuable insights from this enormous dataset, overcoming the challenge of data volumes.
Machine learning for PPC optimisation:
Technical challenges overcome: data velocity, data volume
For clients who have a large product set, data science techniques relating to anomaly detection and machine learning can prove incredibly useful in overcoming data velocity and volume challenges. The data science team can develop a system to help clients quickly identify rising search queries that are triggering ads, but not matching products on-site. Using a query velocity prediction for each matched keyword, the system identifies when an ad is triggered more than it should and then sends this via an alert to the PPC team who can determine whether this rising search term should be added or excluded from the account. In some accounts, this system is checking hundreds of thousands of query velocities at any time and reacting within minutes, overcoming the challenges of handling and deriving actionable insight from a dataset with great velocity and volume.
Speaking from personal experience, these methods are tried, tested, and proven to be effective in helping brands understand and be able to make use of the mass of data at their disposal, remove silos and increase the effectiveness of their performance marketing activity.
Follow ExchangeWire