To Extract Value from Data, First You Need to Understand It
by Lindsay Rowntree on 8th Mar 2016 in News
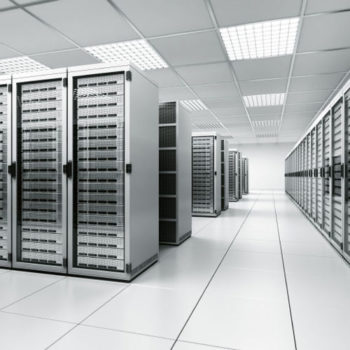
There is a lot of hype around data and how you can extract maximum value from it. But hype often leads to fear of the unknown, resulting in vast quantities of data, from different sources, each source providing a varying level of value. In the first of a series of pieces exploring data, Mathieu Roche (pictured below), global business development director, Weborama, explains the difference between data creation methods and how they can be put to best use.
Marketing has become a scientific, data-driven practice. It has taken 20 years since the early days of the internet and the promise of one-to-one targeting at scale – and more than 100 years since John Wanamaker famously expressed his frustration of wasting half of his marketing spend – but we are finally there. Thanks to the programmatic infrastructure, data can now flow freely between its origin, website, applications, CRM databases, and its destination, a digital touchpoint with a prospect or a client. Programmatic advertising is certainly not the only way to use data; but it has democratised the use of data, making it available to most advertisers and publishers, and triggering a wave of innovation around the collection, organisation, and activation of data to improve media and marketing actions.
The learning curve is steep for most 'traditional' advertising professionals who are used to working with broad audiences derived from panel observations. And, as in any learning process, people tend to take shortcuts and hang onto simplistic notions that do not necessarily convey the complexity or the potential of things. When it comes to data, many in the market firmly believe in a limited value system that would crown recent, declared first-party information as the gold standard of all things data-related. This is far from being a universal rule.
Let’s look at data creation methods first. When it comes to how data is created, you can identify three different ways: declaration, inference, and modelling.
– Declared data is provided voluntarily by users, when filling in a form or answering a questionnaire. Declared data usually relates to demographic characteristics (who they are, where they live, how many kids they have, how much they earn, etc.) but can also provide information on their interests (what kind of sport they like, where they would rather go on holiday), media habits (what channel and programs they watch, what newspaper they read) or even purchase intent (do they plan to buy a new car in the coming year, or take a subscription at the gym). To use a real life analogy, declared data would be what a customer tells the store clerk about them when they enter the shop (“Good morning, I am looking for a pair of sports shoes for my five year-old”).
– Inferred data is derived from users’ behaviour, usually observed online or through their purchase history. If someone spends a lot of time on a golf news website, it is fair to think that they play (or at least enjoy watching) the game. Browsing home improvement pages? DIY enthusiast. Has recently purchased a pushchair? Likely pregnant or just had a baby. Reading about the upcoming iPhone7 release? Interested in new technologies. You get it. In the real world, inferred data comes from the observation of a customer’s behaviour: if you are looking at the family sedan in the car dealership, the salesman will probably make assumptions about who you are and what you are looking for, inferring that you are a family man in the market for a car.
– Modelled data leverages statistical methods to predict the behaviour of a population based on the behaviour of a sample of users. Various data modelling methods (algorithms) can be used, all with more or less exotic names (logistic regression, segmentation tree, random forest, etc.) but with the same end goal in sight: to identify explanatory variables and the best method to combine them to predict an outcome. In real life, modelling would mostly rely on experience. Because travel agents know that cruises are very popular with senior couples, they would focus on these types of products first when engaging with senior prospects.
Out of these three creation methods, surely declaration is the best, or at least the 'truest'. Well, not necessarily. To put it bluntly: people lie. There is a much bigger share of people from Afghanistan (usually the first country in the dropdown list) in survey respondents than in real life. And consumption of high-end media, such as BBC Radio or French newspaper Le Monde, is much higher than their actual circulation. It is only human nature to try to embellish the image we give of ourselves, while we really just want to watch the X Factor and read the Sun! From a marketing standpoint, declared data often provides a skewed perspective of who people are, what they do, and what they want.
More importantly, declared data can only go as far as the information people are willing to give away. When it comes to discovering value, potential, risks, or opportunities, relying on statistics is a much better method. Product recommendation is an easy enough illustration of the potential of statistical data creation: because one knows that clients of 'product X' usually end up buying complementary 'product Y' after a few weeks, they can promote it aggressively in their upselling effort. Even though the need for 'product Y' has not be expressed (declared) by the clients, inference tell us that its sell-through rate will be higher on 'product X' clients that on the rest of the population. In a similar fashion, data modelling is a very efficient way to create scalable value from a limited data set. Looking at a sample of high-value clients extracted from their CRM database, marketers can identify variables that are explanatory or predictive of future value in a prospect population, and act upon them.
Data inference or modelling require statistical methods to be applied: one must work with a large enough, statistically representative sample of users to make sure their analysis is not skewed one way or another. It is important to consider positive, as well as negative, examples to understand what makes populations different. Always take into account margins of error in your analyses, to focus on statistically significant improvements only. And last, but not least, use a large and granular enough projection database to make sure that the outcome of your modelling efforts are both powerful and scalable.
When done right, inference and modelling are the best way to uncover hidden value in a dataset. These data creation methods are not necessarily better than declaration, but they provide a different, complementary view of a population. So, don’t rely on so-called 'common sense' to assess the value of data. Instead, focus on the methodology and on the business application to determine which method is most appropriate for your needs.
Follow ExchangeWire