High Ad Fraud Figures are Scare Tactic: Q&A with Dr Felix Badura, Co-founder, Meetrics
by Lindsay Rowntree on 10th Mar 2016 in News
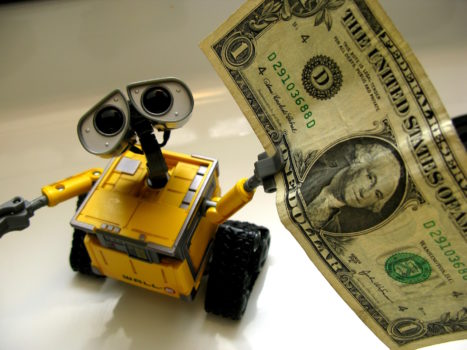
Following the recent JICWEBS town hall event, which focused on the core issues of ad fraud, brand safety and viewability, ExchangeWire speaks with Dr Felix Badura (pictured below), product director & co-founder, Meetrics about the prevalence, detection, measurement, and impact of ad fraud.
ExchangeWire: There are a lot of estimates flying around about the prevalence of ad fraud – what figure is your money on?
Dr. Felix Badura: There’s been a tendency to scare the market with increasingly higher estimates – which suits certain self-interests. Recently, for example, a major ad-serving company CEO opened his keynote by saying 64% of US ad requests are non-human traffic. In reality, we see an average share of fraudulent traffic around 3% in all markets. However, individual placements can, in some cases, be subject to almost 100% suspicious traffic. The figure, of course, also differs greatly between programmatic buys and direct buys.
Other than prevalence, has fraud developed in any way over the last year?
The main way has been the debate evolving from the estimated prevalence and cost of fraud to how to combat it. The work of industry bodies, such as the Trustworthy Accountability Group and JICWEBS, who produced a taxonomy of fraud categories, has supported this process. Furthermore, both agencies and publishers have increasingly started to analyse their own traffic and processes in order to identify and eliminate sources of fraudulent impressions.
What's the best technique to detect fraud?
Fraud can be bucketed into two categories: either the page itself loads ads in a fraudulent way, or the ad is correctly displayed but the viewer isn’t human.
Thus, to detect fraudulent ad impressions, a snippet of JavaScript code should be added to the ad creative or the publisher page. This code analyses relevant page and browser attributes which are transmitted to the Ad Verification vendor’s server. To then classify an impression as fraudulent, a variety of checks should be applied; focusing, for example, on inconsistencies in the rendering behaviour of the browser, the origin of the user (e.g. is the traffic originating from a data centre instead of a residential IP address) or the page layout. Besides these checks, which focus on the individual ad impression, patterns that appear across several impressions are also a viable source to detect unrealistic behaviour.
What are the main factors that impact the fraud rate?
There are three of them:
- The price of the ad: the higher the yield placements, the more attractive they are to fraudsters (e.g. video deliveries).
- Delivery chain transparency: the less transparent, the harder it is to narrow down at which location in the ad delivery chain the fraudulent behaviour occurs. This is especially the case in open-exchange inventory, as it’s often forwarded from one party to the other before it’s actually sold to an advertiser.
- Video ad tracking standards: some are more susceptible to fraud than others. The most common, VAST quartile trackings, is more limited in how fraud can be detected. It evaluates the number of ad deliveries and the completion rate (how many ads technically reached 100% of the ad length), but it’s easy to simulate these events, for example, by calling the required pixels in a predetermined order out of a data centre. More sophisticated tracking mechanisms, such as VPAID, are less susceptible to fraud.
What are the greyest areas around what is and isn't fraud?
JICWEBS identified “16 different malicious (possibly fraudulent) and non-malicious sources of non-human traffic; but this isn’t an exhaustive list”, so this gives you an idea of the scope of the debate. Certainly, not all of these would classify as fraud in a legal sense.
However, it shows how important it is to specify, on insertion orders, more precisely what an advertiser expects from a certain publisher, especially with regard to borderline cases.
For example, viewability or ad density (sometimes also referred to as ad clutter) are very meaningful metrics when it comes to judging the quality of a placement. Ad deliveries that aren’t viewable, however, are not necessarily fraudulent. Also, with regard to ad clutter, many advertisers might – in exchange for a lower price – accept that their ad isn’t the only one on the page but next to three or four other ads.
Another grey area is the automatic reloading of ads. If publishers are selling ads on page impressions that last 30 minutes (e.g. next to a video player) it seems legitimate that ads in these slots are reloaded after a certain time. If the reload happens every five seconds this won’t often be tolerated by advertisers that expect a premium ad delivery.
Allied to this are automatic page reloads, particularly popular among French publishers. Publishers tend to declare this practice as a courtesy to the user, who can open the page in the morning and see the most updated version every time he returns to the tab. A nice side effect, however, is inflating impression numbers. As a result, JICWEBS classifies this practice as “potentially” fraudulent and ad deliveries in inactive tabs will, anyway, never be counted as viewable impressions.
Another very grey area is when video ads are delivered in small 300x250 pixel slots. While this might be a legitimate practice, many deals explicitly ask for in-stream inventory in order to avoid this kind of delivery. In response, some video networks have started to buy small ad slots, in which they initially load editorial video content that automatically starts playback. After a few seconds, this video stream is then interrupted by a mid-roll ad spot. Since it is now part of a stream, it might be considered as an instream ad, even though it is running in a container that one wouldn’t associate with video ads.
JICWEBS' intention, as with brand safety and viewability, is to certify vendors who claim to detect/measure fraud. What are the main challenges this faces?
Compared with viewability, fraud is much harder to measure – there are different definitions, far more grey areas and it’s an ever-changing entity.
For example, to validate if a vendor can detect invalid traffic from a botnet, an auditor needs to simulate such practices and mix it with real user-generated traffic in order to let the vendor separate them again. Unfortunately, botnet providers invest a lot of time in stealth techniques to disguise their activities (e.g. by declaring them with a forged user agent). As a result, various different tricks are used by specialised vendors to identify, for example, whether a certain browser acts as expected under different scenarios. To create a fair test scenario, that also gives the market an overview to which extent bot tests are developed, an auditor, therefore, would ideally have to use several different bot types at once.
This is extremely hard, compounded by further difficulties around some cases that rely on big data analyses by the vendors (e.g. cross-campaign analysis), which make it hard to simulate behaviour in a closed environment.
Ad FraudBrand SafetyDigital MarketingDisplayRegulation
Follow ExchangeWire