Inferred vs Observed Data: Do You Really Know the Difference?
by Lindsay Rowntree on 22nd Mar 2016 in News
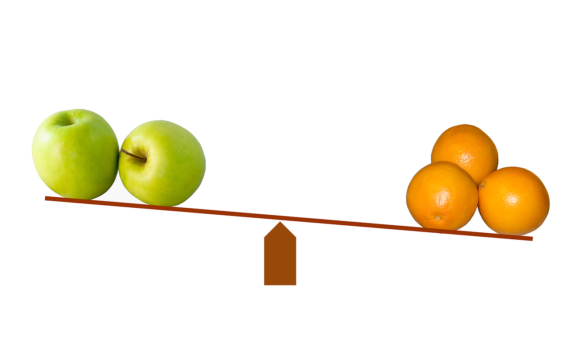
Data has become a fundamental component of our lives. Every decision we make online is recorded and stored for future use. The more we do online, the more data there is on our habits and preferences, from the pair of shoes we are saving up to buy, to our favourite holiday destination. For brands, the more data you have on consumers, the more accurately you can target them, leading to a better user experience and greater return on investment. Edward Thomas (pictured below), head of audience, Skimlinks, explains to ExchangeWire that with so much data available, it’s hard for brand marketers to understand which type of data will make the difference. Thomas tells ExchangeWire five things to consider when buying targeting data.
Without in-depth knowledge of the space, it can be easy to buy data that is not properly substantiated and invest in the wrong area. When considering what data to use, it’s important to understand the different types of data available and the right questions to ask to get the best results.
What is high-quality data?
In targeting, determining whether the quality of data is either ‘better’ or ‘worse’ predominantly rests on its predictive capabilities. However, what you are trying to predict will also affect the quality. For example, if you’re interested in what someone will read, looking at what they’ve read before is a good bet. But, if you want to know what someone is likely to buy, looking at just their age, gender, and reading habits is not enough to make an accurate prediction. You also need to know their shopping history, frequency of purchases, typical order values for categories of products, and brand preferences.
As a rule of thumb, data sets should be built using information that has been gathered from users performing the action that you are trying to predict. So, if you’re targeting shoe shoppers, choose segments from a data provider that can observe large numbers of users buying shoes to give you the best predictability. A data provider that has seen one billion users go through a process will know what steps precede a certain action, and will allow you to target the right users at the right time.
How should data be gathered?
You can learn the most about consumers’ behaviours and habits through direct observation. It is far more reliable than self-reported intent, which can result in fallacies. For instance, a user may intend to buy an item, but be unable to afford it. Similarly, users tend to underestimate their likelihood of making impulse purchases. Only by observing a person's actions across a statistically relevant time period can accurate predictions be made.
What challenges are there with direct observation?
Data that has been gathered purely through direct observation has not been altered by processing or combining different data sets, and comes from a known, relevant source. However, the challenge is having enough volume and variety of data points to make statistically relevant predictions. For example, only tracking a user at the point of purchase will give you little information about what led to the purchase decision in the first place.
What is inferred data and how will it help me?
Any prediction is an inference; and the process of predicting future behaviour from past actions is the backbone of targeting with data. However, to maintain accuracy, the raw data has to be real observed behaviour. When it is not, and the predictive modelling is run on inferred data, the result is a prediction based on other predictions, exponentially lowering accuracy to the advertiser’s detriment. Watch out for this, as some data providers can use this technique to increase the volume of user IDs they can target.
When choosing a data provider, it is important to know how much of their data is gathered through direct observation and how much is inferred. If you’re looking for accurate targeting, the answer should be 100% direct observation.
Why is data validation important? How does transaction data help?
There are two types of data validation. The first is the validation of raw data, which checks that the data sources and the processes through which the data is gathered are transparent and relevant. The second is the validation of predictions. When buying audience segments, you are paying to target someone who is predicted to have a certain behaviour. Unless the success of these predictions is validated, there is no way to know how accurate the targeting will be; and, therefore, if the return will make up for the price paid.
This means the provider must have data points from both ends of the funnel: the actions preceding the activity they are predicting, the moment the action took place in practice, and the steps that followed. For instance, any provider that claims to predict shopping intent must have access to transaction data to validate that a purchase took place because of targeting.
If you follow these tips, you should end up with data sets that allow you to target the right consumers for your brand at the right time.
Follow ExchangeWire