Let Machines Do What They Excel at: Q&A with Peter Silverwood, Chief Scientific Officer, IO
by Lindsay Rowntree on 20th Jun 2016 in News
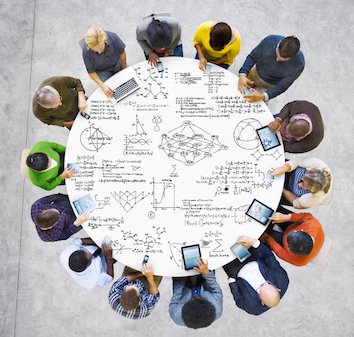
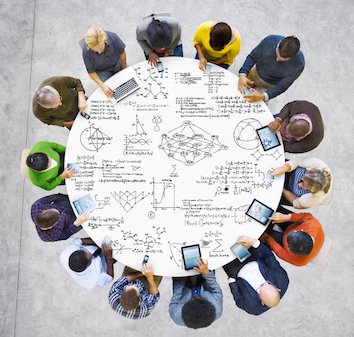
The UK DSP market is a tough market to operate in, let alone launch in. Still a relatively new kid on the block, and founded by an ex-Met Office employee, Intelligent Optimisations (IO) are making a name for themselves in an, arguably, saturated market and flying the flag for machine learning. Following his recent promotion to the newly created role of Chief Scientific Officer, IO, Peter Silverwood (pictured below) explains to ExchangeWire exactly what that title means in the DSP world.
ExchangeWire: As recently promoted Chief Scientific Officer, what does your role at IO entail?
Peter Silverwood: My role covers two distinct but complementary areas – ‘performance and machine learning’.
The performance part is operations-based, working alongside our campaign delivery teams to constantly monitor and optimise against client targets. We test alternative algorithms and build out strategies for each customer.
On the machine learning side, I manage the important and successful algorithms and transform these into platform capabilities. Sometimes these are approaches that come from research, sometimes industry specific knowhow. I have the responsibility to use that industry-wide knowledge to make sense of extensive amounts of data, identify patterns and sub-optimal processes with room for improvement, and forecast any potential opportunities.
My main focus at IO is on developing the learning aspect of the platform in a way that delivers on our customer’s needs.
Chief Scientific Officer roles are largely reserved for companies operating scientific research – how is that relevant to demand side platforms and the wider programmatic ecosystem?
IO is a ‘digital-first’ platform; so big data and data science is engrained in the way we work. We are really marrying programmatic with machine learning to deliver against campaign targets and often exceeding them. We’ve seen a 90% improvement in CPA, on average, across all campaigns from week one of campaign launch to end of month three, and these efficiencies keep on improving.
Our team have built a complete demand-side platform from the bottom up that is able to analyse the complete bid stream from the ad exchanges with real-time data and a complete set of profiles. All of this data is processed by multiple sophisticated algorithms, running in tandem, assessing and assigning a value to each opportunity to identify intent and bid appropriately within microseconds.
Our cutting edge machine learning ensures we deliver not only the best performance for our customers, but also enables us to provide full transparency, through clear, actionable insights from the learnings, presented in real time throughout the campaign.
The scientific part of our media buying is encapsulated in IO’s methodology, that supports these learnings on effective media spend patterns and programmatic performance with robust statistical and scientific good practice. For me, this is key to pushing forward the boundaries of programmatic value.
As Chief Scientific Officer, you're being touted as a 'Machine Learning figurehead' within IO – what does this mean?
Simple: evangelise the application of machine intelligence, our key competitive advantage as a next generation technology company, in everything we do.
After spending the last two years creating the platform with a 40-strong R&D team based in the UK, we are now able to provide a very granular level of insights on how a customer’s campaign has performed. This is a constant learning and improvement process with everyone at IO pushing the boundaries of technology and applied research to seamlessly improve our delivery targets and performance, providing our partners with unparalleled levels of transparency and, therefore, building trust.
How is data science changing the face of ad tech and programmatic?
Gone are the days of John Wanamaker's quote: “Half the money I spend is wasted; the trouble is I don’t know which half”.
We find motivated prospects by identifying intent, and data science is a key element in this. Once a marketer begins to consider context, or the macro-environment, they quickly stand a chance of making an impact right at the bottom of the marketing funnel, the point of transaction.
Buyers are giving off more intention indicators than crude product searches, if you know where to look. That means monitoring for behaviours, connections between online activities, referencing against historical buyer patterns and leveraging in-the-moment data in the process, something that requires sophisticated technology. The application of data science enables us to make sense of it all.
The fundamental premise of programmatic is visibility and accountability for all spend, and data science underpins being able to achieve that. Transparency across how media is bought and optimised means that marketers can be closer to real-time media decisions at all times and, therefore, able to adjust and align to key business goals.
What role can humans play in a programmatic ecosystem that is ever-reliant upon machines?
I don’t buy the idea that machines will take over from humans; but what is sure is that they will change the way we work.
Machines are able to learn from billions of data points within a data set in fractions of a second, relentlessly adjusting and optimising in a way that humans cannot; whereas, only humans can draw on experience and associations from outside the bounds of a data set presented to them. Advertisers will need both machine learning and enduring human planning capabilities, so one is not a threat to the other.
It’s actually a hugely positive development in my eyes. We should let machines do what they excel at – running options, working out media planning at the granular impression level, and automating cumbersome processes. This, then, allows us to focus on tasks that can create real value: offline modelling, challenging their brief, and doing more of what we are best at – applying experience gained outside the confines of a defined data set and, of course, extending and improving the machines themselves.
Follow ExchangeWire