Algorithms Are Smarter than People When Catering to Customer Intent
by Lindsay Rowntree on 3rd Aug 2016 in News
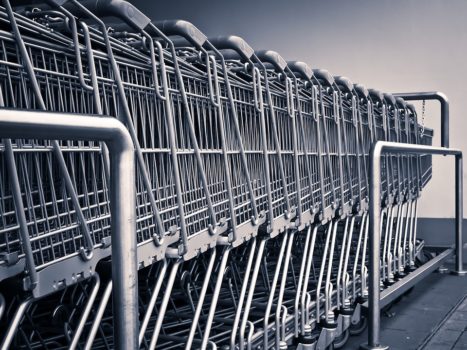
Do marketers know what their consumers really want? If they think they do, on what are they basing this knowledge? With so many fragments of customer data, can a brand really be certain of the intent of its consumers? Tom Bowman (pictured below), CEO, Intelligent Optimisations writes exclusively for ExchangeWire, where he argues the understanding of how a marketer can cater to a customer's intent may be more complicated than expected and, ultimately, empathy with what consumers want holds the key.
If I said to you, intention is the cognitive representation of a person's readiness to perform a given behaviour and is considered to be the immediate antecedent of behaviour, you may understandably scratch your head.
In fact, this is a well known concept that has been used in marketing for years. Ajzen and Fishbein’s 'Theory of Planned Behaviour' simply holds that the best predictor of behaviour – which is, of course, a useful targeting indicator – is intention.
That is, a consumer’s intention to perform a task, buy a product, or visit a place, is the thing that makes it most likely you will score a positive hit from a marketing message.
Over the years, marketers have used some pretty crude and sometimes exotic proxies for locating consumer intent. Today, brands operate a CRM approach to targeting customer intent. We are sitting on vast repositories of data, which we can turn into information and, subsequently, insight to estimate a consumer’s next action.
For example, BMW may say that its best customers are not new ones but its existing ones. It is useful to know who existing owners of BMW 3 Series are and for how long they have owned the vehicle, in order to try upselling a 5 Series or a brand new 3.
When you buy in third-party data sources through a data management platform (DMP), you can easily augment the customer profile to gain a fuller picture. In truth, however, this is just half of the promise of programmatic advertising.
This kind of decisioning is entirely predicated on mining the breadcrumbs dropped by consumers in the past. But what people did isn’t necessarily what they are going to do next.
Knowing your BMW customer isn’t necessarily going to help you if you are only guessing, using customer satisfaction surveys, that they want to stay in-brand or move to a higher-end model. What if your BMW-owning bloke loves the 'ultimate driving machine' but, now a dad, is looking for a more practical vehicle?
If you don’t already hold that historic but brand new profile data, you could lose the customer. But responding to his customer intent, at the very time he is seeking out a new car, holds the promise of better results.
Imagine your customer is in a supermarket, walking around the building, from aisle to aisle, assembling items into tonight’s prospective dinner party meal. Now, imagine instead, you moving the relevant aisles right in front of them, like Yoda using the Force, at exactly the moment they needed the next item.
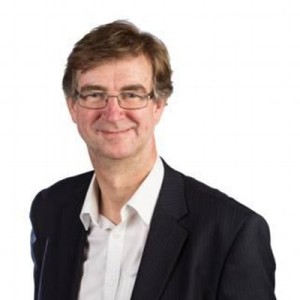
Tom Bowman, CEO, Intelligent Optimisations
That is what it means to cater to customer intent. This may be hard for many marketers to understand, but, with programmatic advertising, when you can see a consumer’s past and recent behaviour, you have the option, not simply to make an assumption about intent, but to cater to it as it is seen.
Algorithms are smarter than people at divining this behaviour. Which is a good job, since, to process the many customer data fragments and cross-reference the many disparate data sets, with appropriate weighting to key inputs, in order to deduce a customer’s likely intention… this is brain-busting stuff of the kind only machine learning and artificial intelligence can help with.
It doesn’t take a rocket scientist to predict that ice cream ads work well when the weather is hot. But understanding the difference between one multi-part set of circumstances (it’s raining, a customer is at home, and the economic forecast is bleak) versus another (the sun is shining, England just won the Euros, and a customer is in the park) – that stuff is as hard as it is transformational.
However, there is some important groundwork brands can put in today:
1. Separate key metrics
In programmatic, many marketers combine data for prospective customers with those for reaching via retargeting. This means they never separate out the search for new customers from simply hitting known targets over and over. To harness intent, raise the Chinese wall between these two data sets.
2. Separate platforms
Just as intent-based ad buying is a different paradigm, so it requires different technology platforms. So, don’t try and shoehorn one practice into another – pick technology that can make the most of intent-based targeting as distinct from other practices.
3. Separate strategies
Programmatic is not just a single thing anymore. What used to be thought of as simply automated bidding from auctions is now several things. Programmatic has now gone up to the top of the marketing funnel. So, think of the practice as catering distinctly for branding, prospecting, and targeting to make the best of each.
4. Demand insights
It is not a dark art; in fact, it isn’t an art at all. Accessed programmatically, the intention in consumers’ actions is the product of science, and the tracking of it is, too. You should expect that partners report back on the science of your campaign’s achievements.
Consumers’ intention really holds the key to the marketing conundrum of 'right message, right person, right time'. It means ensuring that, when you find a new mum browsing Mumsnet at 2am, your systems don’t show her an ad for a product sale with a checkout on the other end – chances are, she is not holding her debit card. It means picking an alternative and returning the following evening.
What it boils down to is buying with empathy for what consumers really want.
Follow ExchangeWire