Marketing AI Needs to Change to Live Up to the Hype: Q&A with Brett House, Nielsen Marketing Cloud
by News
on 7th Dec 2017 in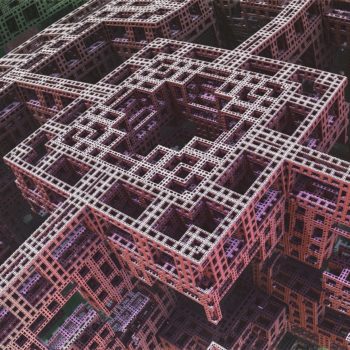
Artificial Intelligence (AI) is everywhere these days. But is it just new marketing lingo added to something that been around for years, or is it truly something new that can make a difference in how marketing is performed? Brett House (pictured below), VP of global product marketing, Nielsen Marketing Cloud, talks about the hype, the reality, and the potential of AI solutions in marketing and advertising.
ExchangeWire: How do you define marketing AI?
Brett House: AI is a loaded term – and one that has been wildly overused in the marketing technology and ad tech space. So much so, that eMarketer just released a report saying nearly half of all marketers think it’s overhyped. And, in many cases, it is. What a lot of companies call 'AI', is really just the same batch-learning-based modeling data scientists have been doing for years with a new name.
To live up to the hype, marketing AI needs to move beyond batch-learning. It needs to employ a self-learning system that’s plugged into a constant source of customer information, like CRM or point of sales data. It should also automate those time-consuming data science tasks – like model training, monitoring, and optimisation.
The problem is, most of the so-called 'AI solutions' currently available aren’t self-learning systems. They don’t automatically identify and adapt to what consumer attributes and behaviour are driving campaign KPIs – like form fills or sales conversions. For marketing AI to work, new models need to be automatically built, trained, and launched with little to no marketing or tech involvement. Most available 'AI' modeling solutions take days, even weeks, to get off the ground, and don’t scale very well to handle the complex marketing needs of big companies.
How many AI solutions live up to the claims of being real-time?
Very few. Honestly, most AI currently on the market employs batch – or offline – learning. These solutions, as the name implies, are slow. The only thing that’s fast is the act of scoring segments, which isn’t nearly enough to help a marketer’s ability to act quickly and decisively on changes in audience behaviour.
So, say you are a retailer running a Cyber Monday sale using a batch modeling approach to power your paid and owned audience targeting during the period, which is pretty standard practice today. First, a couple of weeks in advance of the holiday, the retailer’s data science team (or that of a vendor) will need to pull all their first-party data – such as CRM, point-of-sale, and media engagement metrics. This information is analysed in an offline setting via a computer, where the models are trained (on what to do) and then finally deployed. This process can take over a week. All the learning is happening offline based on old data.
The problem with this approach is obvious. Shopping behaviour changes at the blink of an eye throughout the holiday shopping period. People comparison shop, search for deals, respond to offers, read reviews, and move in and out of market for products as they buy things. Those Cyber Monday models the retailer launched won’t capture any of that behaviour. They use historical data and have no ability to update on their own once they’ve gone live. It’s a static approach to a dynamic problem.
The dynamic approach to AI is something data scientists call 'online learning'. Simply put, online learning has a built-in self-testing and correcting mechanism that adapts to changes in the data it sees and gets more accurate over time. So, if a customer makes a purchase, clicks on a search ad, or abandons a shopping cart, it learns and adjusts accordingly. The learning is happening online versus offline, so it reflects current realities. And, importantly, the models are evergreen, so they actually learn and adapt, which is huge when you're a marketer dealing with hundreds, if not thousands, of live models at any point.
How do marketers see into the blackbox to understand, and even adjust, what these AI systems are doing?
That’s a really good question. It really comes down to a self-service user interface that makes the management of advanced machine learning something anyone on your team can handle. Data scientists and technologists shouldn’t be required.
Nielsen AI clients, for example, have AI transparency into what their models consist of and how they are performing. They can blacklist consumer attributes they don’t want associated with a model and whitelist those that they want the model to focus on.
We think it’s imperative for our clients to understand what’s impacting model performance and to have some control over it. They can see what’s happening beneath the hood in a really simple way, and aren’t forced to merely trust what the 'AI brain' is doing, which, to the average person, is pretty daunting.
What does this mean for advertisers?
The right AI solution is like having an army of data scientists and a warehouse of servers at your fingertips. The ability to instantly build and propagate new audience models in a way that would historically require a large team working around the clock means not only cost savings, but also more responsive and optimised advertising and content at a huge scale. This should translate into less advertising waste and better customer experiences.
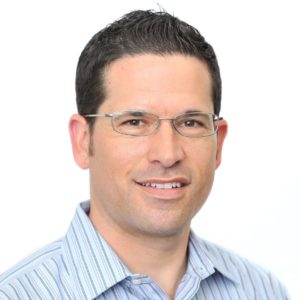
Brett House, VP of Global Product Marketing, Nielsen Marketing Cloud
Imagine a real working situation: your team tells you that specific combinations of audience targets and digital channels are resulting in big jumps in conversions for a recently flighted campaign. You want to double-down and optimise towards these combinations; but it’s 5pm on a Friday afternoon and most of your team is leaving for the weekend.
With the right AI-powered software in place, you can go home and rest assured knowing that models will be automatically built that identify what consumer attributes and behaviour are driving these conversions. It will also activate these newly optimised audience models across all of your marketing execution platforms, such as email, your website, programmatic, mobile, and OTT-TV, without requiring your team to do anything. This ensures you are meeting the needs of the people most likely to convert at all times and in all digital marketing places, regardless of where your team is.
How can publishers – or media owners – use AI?
One of our most successful AI implementations is with a large digital publisher in the financial services space. They use Nielsen AI for content optimisation, which in their case means updating content based on how their audiences are behaving as well as the impact of rapidly changing financial market conditions. They have nearly a hundred digital properties; so this takes automation and processing power, not to mention high-quality online and offline data, which they are using to great avail.
This content-optimisation approach is applied to their client’s advertising campaigns so they can better target and personalise the ads they show across their properties. They have seen big jumps in their clients' campaign performance across the board as a result.
They are also using Nielsen AI to better price and package their inventory. It analyses multiple data streams, like website traffic patterns, offer interest and intent, viewability, and content engagement to help them more dynamically adjust CPM rates. This is a great example of how AI can power predictive analytics. It helps them predict performance, which enables them to justify different prices for different audiences and content.
Follow ExchangeWire