Successful Audience Modelling: Retail Data as a Catalyst for Creativity
by Hugh Williams on 14th May 2018 in News
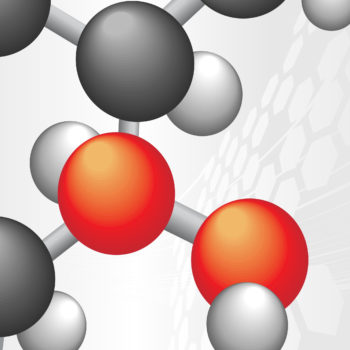
In this piece, Lars Engelbrecht, director, ad operations and solutions, Retail Media Group (pictured below), tells RetailTechNews how advertisers have increasingly come to understand that it generally makes little sense to roll out campaigns using a scattershot approach. Pure branding campaigns that work via wide reach and broad slogans following the one-size-fits-all principle usually suffer from substantial scatter loss. Nevertheless, the larger the database, the more information can be gathered about target groups and more interesting audiences can be generated. One of the most promising proxies for the interests and needs of every target group is retail data and, thus, insights into which products have actually been purchased. But a promising database alone is no guarantee for successful campaigns – so, how do advertisers model the right audiences and what do they have to pay attention to when addressing their target groups?
Audience modelling as an ongoing process
There are several ways to define audiences. On the one hand, advertisers can analyse their target groups in the run-up to a campaign, i.e. pre-campaign, and model their audience accordingly.
Essentially, they make an assumption and try to validate this assumption in a targeted manner. Another option is to analyse target groups during and after the campaign – in this case, the audience-modelling process runs concomitantly alongside the campaign.
Basically, an audience should not be rigid. Therefore, regular anonymous analyses are essential for the success of a campaign. It is not advisable to rely solely on pre-campaign analyses; the audience must also be constantly monitored and fine-tuned during the campaign.
For instance, if advertisers want to promote a high-priced product, it would appear obvious at first glance to target audiences with large shopping cart sizes and high purchasing power and frequency. But there are also many other factors that one would not initially suspect to be relevant. Often, data that is supposedly less relevant only provides crucial information about a target group in correlation with other findings. For example, seemingly irrelevant information such as time of day or weather can have an impact on purchasing behaviour and, in combination with the size of the shopping cart, can provide valuable insights into the audience.
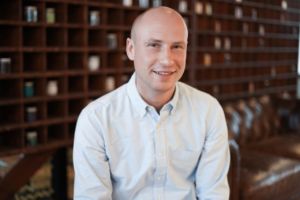
Lars Engelbrecht, Director, Ad Operations & Solutions, Retail Media Group
Hence, advertisers need to regularly ask themselves: What is it that characterises my target groups? In which shops do they purchase products? Which brands are they interested in? What other, supposedly non-relevant, information can I find out about my target group? Based on these considerations, an anonymous analysis is carried out that evaluates the statistical relations between various actions of the target group. Using machine-learning algorithms and methods to identify key correlations, recommendations are then made to create new audiences. These newly defined audiences must also be refined during the ongoing campaigns. If the newly identified users have a similar purchasing behaviour to the target group who has been addressed so far, there is a high probability that they will also become customers.
Audience modelling must, therefore, always aim to reach new audiences who could potentially be interested in the promoted product.
Data vs creativity
A good database alone does not make a successful campaign. Data must always be the catalyst for creativity – only when advertisers know what characterises their audience can they know which messages, visuals, and graphics are best suited to address them. Certain audiences react to certain stimuli – bargain hunters have to be addressed with other messages and creations than do luxury shoppers. Therefore, audience modelling and creation should go hand in hand. To ensure that this is the case, A/B testing must be used to determine which creation fits which audience. These results, in turn, provide information on the preferences of the respective audience. More often than not, there are two or three interesting ‘outliers’ that advertisers did not expect. Insights such as buying behaviour and touchpoints can be used to check whether the frequency of an action over a certain period of time is just a random occurrence. This type of ‘outlier’ can then either be verified or refuted with a new campaign. If it is verified, the advertiser should consider: What does this mean for my campaign? Would it be advisable to set up suitable promotions, offers, or discounts? If audience, targeting and creation are all in alignment, there is nothing standing in the way of a successful campaign.
GDPR, ePrivacy – and what now?
The legal data protection framework for data collection and processing must be adhered to at all times in order to continue the programmatic and target-group-specific delivery of campaigns. How exactly the future development of audience-specific advertising in the context of regulations such as GDPR and ePrivacy will unfold is yet to be seen. From the consumer's point of view, any regulation providing information on what happens with their own data is more than welcome. After all, users should be aware at which point they are actually providing sensitive, personal data and at which point data transmission is non-critical. Even though there is no hard and fast formula for this (yet), one thing is certain: creative approaches to addressing audiences will continue to determine the success of campaigns. Therefore, audience modelling will not become obsolete in the future – in fact, the opposite is the case.This content was originally published in RetailTechNews.
CreativeDataE-CommerceTechnology
Follow ExchangeWire