Using Data Science to Understand the Quantity & Diversity of YouTube Content
by Lindsay Rowntree on 29th Aug 2018 in News
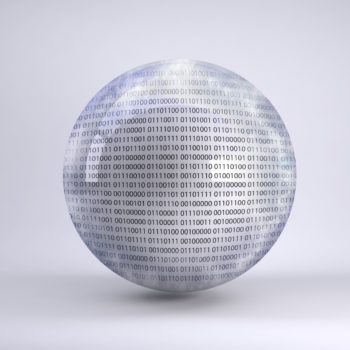
Data science has increasingly been at the forefront of developments in advertising and marketing technology; and with very good reason. In the video space, data science is a crucial element for ensuring brand objectives are met against billions of data signals. In this piece for ExchangeWire, Dr Jon Morra, vice president, data science, Zefr, details the three components of data science in delivering those objectives for brands advertising on YouTube.
We believe that advertisers and brands should be able to place their video ads in front of relevant content for their audience. This is a uniquely difficult challenge on YouTube, the most dominant and still rapidly growing video platform in the world. Unlike traditional publishers and TV networks, YouTube’s explosive growth is due to unlimited content without the constraints of programming budgets and executives, making it an entirely unfiltered video inventory pool for advertisers.
To help our clients navigate this plethora of content options, Zefr builds video-precise content packages, placing advertisers in a contextually relevant content environment. For many clients, this content targeting approach works great. For others, a deeper level of customisation is required in order to truly meet their brand objectives. This is where data science comes in.
I’ve long been a proponent of data science; and as a business we leverage it for growth in many ways. As we focus on custom content solutions for every campaign, our data science work has increasingly come to the forefront of our business. Data science has the ability to manage the enormous volume of signals within YouTube and match those signals with brand objectives, enabling us to determine what is appropriate for each client.
Understanding both the vast quantity and diversity of content on YouTube with data science has three critical components:
1. Human review
Video-level human review is the most important source of fuel to the data science engine. Human reviewers understand the nuances of each and every brand campaign. This allows us to determine that a major luxury beauty client wants videos centred around the application of daily makeup, but not videos about Halloween makeup, which are off topic. This is the kind of nuance that only a human with brand expertise can deliver. When performing reviews, reviewers should make decisions holistically, focusing on the entire video content and metadata combined. This allows data science to tease out patterns in a variety of fields and not just focus on the video title, for instance. When people make decisions holistically, we’re able to find patterns in the data that are much richer than we could get by only reading feeds. To date, we have done tens of thousands of reviews with this brand focus and continue to gather more video reviews every day. Combining this with a deep historical understanding of YouTube content allows for highly precise campaign recommendations.
2. Information extraction
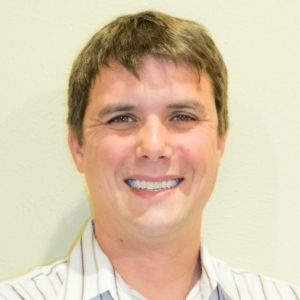
Dr Jon Morra, VP Data Science, Zefr
After gathering many thousands of video reviews, the next key element of the data science process is called 'featurisation'. Featurisation describes the task of taking a complex piece of data, in our case a video on YouTube, and breaking it down into its component parts, each of which are directly understandable by a machine.
Every video consists of numerous pieces of information, including the various thumbnails, the title, the description, the number of views, and the publish date, to name a few. During featurisation, our data scientists pull these pieces of information out of a video and its metadata, and organise it so it can be ingested by pattern-recognition algorithms. Because of the different types of data in a video (image, audio, text, numerical), such learning is frequently called 'multi-modal' learning. Leveraging multi-modal learning allows us to find patterns in many different components of a video and is, therefore, a competitive advantage over solutions that just look at one component of the data, such as text.
3. Machine learning
Once we have enough human reviews, and a featurisation pipeline, we are ready to apply machine learning. Data scientists need access to state-of-the-art algorithms including random forests, gradient boosting machines, and deep neural networks to find distinct patterns within data. By using a variety of machine-learning platforms, data scientists can optimise their choice of algorithm to best suit the available data and the client’s desired outcome. As well as the aforementioned, Zefr uses a wide variety of machine-learning platforms (including Vowpal Wabbit, H2O, MxNet,scikit-learn, and LightGBM, to name a few) to ensure we have the best tools to find any patterns present in our data.
At any given time, we are aware of, and can categorise, three billion videos. Because of this scale, we need both a rapid experimental environment to test new ideas (which include algorithms, pattern detection, etc.), and a robust production environment to deploy successful experiments. Our reviewers are constantly working and refining what is appropriate for each client, creating a fluid data set. It is crucial that we automatically incorporate new reviews in near real time into our machine-learning models. We accomplish these goals using these high-quality open source tools and have contributed to the ecosystem with a paper about our production environment, Aloha.
Brands have the best outcomes when their ads are placed in relevant content environments on YouTube; and that requires a process of constant innovation with data science. This effort requires commitment to continuous support, with improved machine learning and scaled human review, based on brand preferences – something which, for Zefr, has created a wholly unique dataset.
Follow ExchangeWire