Yield Management in a First-Price Auction World
by Mathew Broughton on 1st Aug 2019 in News
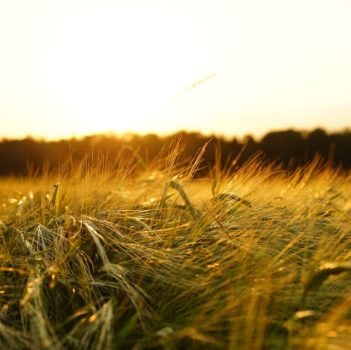
Following the announcement earlier in the year that Google Ad Exchange traffic will transition to a first-price auction model by late August to early September, the industry has been awash with queries as to how this will affect optimisation, and the effect of bid shading tactics.
In this exclusive article for ExchangeWire, Benjamin Hansz (pictured below), vice president of strategy at Simplaex, dispels three of the myths surrounding first-price auctions, and what yield management will look like in these environments.
There has been much discussion and concern across the industry regarding the Google first-price auction announcement, so we thought it a good time to tackle the subject in some detail.
The first thing to bear in mind, is that Google's switch to first-price only affects what happens server-side within Google - i.e. the same call to DFP or last look that they already had before. So, unless Google suddenly manages to gain a tighter grip of overall ad spend, there is no major change in the game for the current players.
Far from being a trailblazer in this case, Google is actually following in the footsteps of what the rest of the industry as first movers have effectively set in stone. The prebid auction already runs on first-price, i.e. everything there remains the same too.
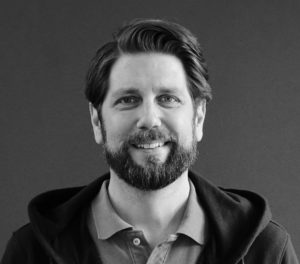
Benjamin Hansz, Vice President of Strategy, Simplaex
If the Prebid auction achieves a higher bid as its outcome, then this also pushes up what Google will need to pay - regardless of which auction type they use server-side.
Essentially, nothing changes.
So, the principal question comes back to second- versus first-price mechanics - and how effective is price flooring when there is no first-price/closing price spread?
Ultimately, what the industry is asking is as follows: what does yield management look like in a first-price world – and does it even exist?
Myth 1: Yield optimisation is only applicable to second-price auctions
Certainly, some algorithms have been set up to the exclusion of all else but second price. Or rather, their machine learning systems have been built purely around price considerations only. Others, meanwhile, have always operated by considering price alongside other factors - user valuation for example, as well as the true ROI of demand. It turns out that this broader definition of optimisation works just as well in first-price environments as second.
Myth 2: Price flooring is no longer effective in the face of bid shading / SPO
Contrary to appearances, bid shading is actually nothing new. It is really just the first-price equivalent of similar tactics that DSPs deployed previously in a second-price context, to pay as little as possible for the ads they buy.
In other words, in both cases DSPs try to gain an advantage by not bidding at their true willingness to pay, but test whether they can push prices down and even sacrifice win rate if it makes their campaigns perform better. In such an environment, the supply side may take their own steps to counter that downward pressure. For example, by constantly testing and readjusting floors to indicate to buyers what they need to bid in order to win - i.e. closing the often huge spread between floor and clearing price, which is otherwise getting exploited by the demand side.
Myth 3: First-price adds unpredictability, constant testing is the only way forward
When it comes to signalling price floors or perhaps flagging other parts of the bid request to buyers to get a better reaction, in first-price just as in second-, this is very far from a random, constant testing process – rather, that testing in time becomes an informed process of learning.
This article describes some good examples of what such a learning activity might consist of. It is also true that random or short-term jumps in floor price don't cause a change in bidding behaviour. On the contrary, how this ends up playing out depends entirely on the bidding strategy and algorithms applied on the demand side at a macro level.
This is why the right supply pricing strategy by no means consists of random price jumps. But rather, with small, incremental changes in the direction which are predicted to generate more revenue. Then observe the results for as long as necessary to be able to assess their impact, before taking the next small step accordingly.
In some cases, it's even desirable not to get an immediate reaction, as it allows the capture of some additional revenue more easily (e.g. by exploiting high bids on a particular subset of users that is missed when only looking on a simple traffic level).
The above article includes a good quote from Shengwu Li, assistant professor of economics at Harvard University. He suggests that setting pricing floors is a question that must be answered with data: “You need to look at the distribution of bids you’re getting, and on a case-by-case basis decide how high that floor should ultimately be.”
That might sound like an impossible task. But in fact, in a first-price world, there is more hope than ever. In the context of the continuing power play between bid shaders on one side, and yield optimisers on the other, it is more transparency that holds the potential for a more sustainable long-term future on both sides - and an end to opacity.
Follow ExchangeWire