The Role of Data Science: Q&A with Kwame Bernard, The Tylt
by Mathew Broughton on 25th Sep 2019 in News
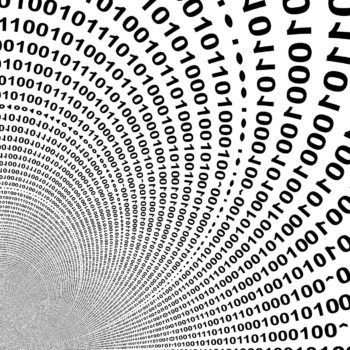
The Gen Z and millennial generations now account for over 60% of the global population, and are seeing rapid increases in purchasing power. These demographic typically expects highly personalised brand experiences, forcing brands to leverage their marketing data like never before.
In this exclusive interview with ExchangeWire, Kwame Bernard (pictured below), data engineer at The Tylt, dicusses how data science can draw out unique insights to help advertisers connect to the consumer.
What are the most effective ways to leverage company data assets to maximise brand value?
IDC predicts that the global datasphere, meaning the world’s collective digital data that we create, capture, replicate, and consume—will grow from approximately 40 zettabytes of data in 2019 to 175 zettabytes in 2025 (with one zettabyte equaling one trillion gigabytes). Trends like data at the edge, continued growth of mobile and IoT devices, cloud and the digital consumer are all contributing to rapid data expansion. From a marketing and business value standpoint, companies are faced with a deluge of data and an unclear path to maximising brand value. One of the most effective ways to maxmise brand value from massive data sets is to engage with customers directly and ask them what they like about brand related topics, in a fun and engaging way. Creating content with a data collection component, like online and social media polling, helps brands to gather real-time audience opinion at scale so that brands can better understand their audiences and foster brand engagement. Data scientists and marketers have a litany of products, tools and services available which have all been designed to understand the customer journey online, but I think asking the audience directly is a unique way to develop insights from a sea of noisy data and build lasting customer relationships.
What different sources and channels can data scientists exploit to create personalised experiences for brands?
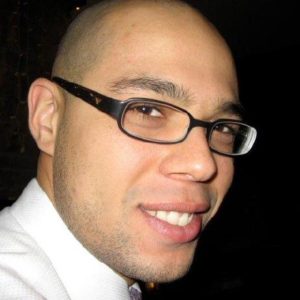
Kwame Bernard, Data Engineer, The Tylt
Social media channels (Facebook, YouTube, Instagram, Twitter, and Pinterest) have evolved from content sharing channels, to ad platforms and are now becoming eCommerce platforms. For example, earlier this year, Instagram launched an in-app shopping feature that allows brand followers to buy products based on the photos they see from their favorite brands and influencers, without leaving Instagram. This move is part of Instagram’s social commerce effort to reduce the number of steps it takes from discovering a product on the platform to purchasing. For data scientists, understanding the path from impression to purchase through new online experiences like social commerce, will allow brands to create more personalized experiences. Opinion polling on a brand’s website and social media channels also gives data scientists more granular data to evaluate and then feed back into branded content experiences. Opinion data is coming straight from the audience and reflects their interests and sentiments.
Are data teams generally being structured effectively to capitalise on personalisation opportunities? Or are they being hindered by traditional siloes?
The digital advertising industry promised a unified customer experience in recent years, but as consumers, we still often receive ads for products that are irrelevant or have already been purchased for example. This is one example of siloed or inaccurate data that can hamper marketing efforts and create friction with customers. Now, companies are being founded with a digital first approach, implementing the software, tools and technologies to eliminate data siloes and promote data democratization. However, many legacy companies not born in the digital era are faced with transforming their existing software and infrastructure – which is a huge undertaking in terms of costs and process.
When it comes to structuring data teams in a digitally native company, data professionals face the pressure to not only gather data and generate business insights, but also to design the algorithms and models that will work best for a brand. This often requires hiring a team of highly experienced data scientists, data engineers, and data analysts with advanced degrees in Computer Science, Statistics, and Data Analytics who can build software, manage big data infrastructure, develop machine learning algorithms, perform complex statistical analyses, and communicate the resultant analyses to non-technical audiences. Understandably, we’re seeing a skills shortage for individual “unicorns” who possess all these attributes with both depth and breadth. While the ideal data professional is experienced with the entire data science workflow, the commoditisation of data and analytics via Data Science as a Service (DSaaS) and Machine Learning as a Service (MLaaS) products provide opportunities for companies and data teams to perform cutting-edge analytics with fewer onsite resources. These DSaaS and MLaaS products lower the barrier to entry, thus encouraging data democratization among stakeholders in an organization while simultaneously allowing members of the data team to focus on their area of specialization (e.g. Data Analytics, Data Science, and Data Engineering) without the need for a “unicorn” data specialist.
Data teams can be a single person in a small organization or can be several people working on different segments: from algorithm design, to tracking and collection, to data processing and analytics. However, having clear objectives is crucial to deriving value from brand data. In data science specifically, companies should be planning for the skills they’ll need in the future to stay competitive.
What are the most prominent challenges when leveraging data, and in measuring ROI? How will these challenges change in the coming year?
The most prominent challenges when using data and measuring ROI are ensuring that the data models, data sources and analytics generate the most business value in real time. Data is often disparate and can be interpreted in different ways by different teams depending on timing. The 80/20 rule is an informal guideline within the data science community that suggests that data scientists spend 80 percent of their time on data cleansing, preparation, and validation tasks while only 20 percent of their time is spent building algorithms, exploring data, and conducting predictive analyses (i.e. tasks that provide the greatest business value). In order to overcome these challenges brands will need to invert the 80/20 rule by structuring their data workflows in a way that automates cleaning and organizing data as much as possible so that the data team can focus on tasks that have the greatest impact on the bottom line. Simultaneously, organisations should promote data driven decision making within the company by casting a wide net of potential stakeholders who will be the consumers of analytics products created by the data team and make sure that those stakeholders are included in the business questions being raised in the first place.
How will emerging technologies such as AI and 5G be used to enhance customer experience for brands and publishers?
One of the advantages of 5G is network-slicing, the ability to use a single physical network to create multiple virtual networks with different characteristics for application-specific use cases. For example, an autonomous vehicle could utilize a virtual low-latency high-bandwidth network slice for mission critical vehicle operations while delivering a mid-tier latency and medium-bandwidth virtual network to entertainment applications for the passenger. In this example, by autonomously managing the virtual network, AI presents a great solution to the problem of increased complexity introduced by network slicing. This convergence of AI and 5G in an exciting example of a technological feedback loop where the AI is being utilized in the new technology (5G) further accelerates developments in AI (autonomous driving). The applications that will benefit the most from 5G are those that require low-latency and high-bandwidth. These include, high-fidelity multi-person video conferencing, real-time gaming, autonomous driving, augmented reality, and virtual reality. These applications will bring with them a deluge of data that AI needs in order to train the deep learning algorithms and artificial neural networks that underpin it.
5G and AI will drastically change the way we share, collect and use data. We’re at the beginning of a new industrial revolution. According to Verizon, “…users will know (5G) as one of the fastest, most robust technologies the world has ever seen. That means quicker downloads, outstanding network reliability and a spectacular impact on how we live, work and play. The connectivity benefits of 5G will make businesses more efficient and give consumers access to more information faster than ever before. Super-connected autonomous cars, smart communities, industrial IoT, immersive education—they all will rely on 5G.”
From a marketing perspective, 5G will process and generate more data than we’ve ever seen creating tremendous opportunities for brands. For example, if a brand is able to present an offer as someone is driving their connected car by their store in real time – that’s a tremendously powerful customer engagement and sales opportunity. All in all, having data that is processed and delivered to its destination instantaneously opens up a new opportunity for brands to enhance customer experiences.
AI will continue to help automate and streamline business processes, freeing up brands and publishers to create increasingly relevant and engaging branded content to deliver to audiences on the right channels at the right time.
Follow ExchangeWire