Data and Analytics: Moving from a One-Size-Fits-All Approach to Customisation and Collaboration
by Grace Dillon on 2nd Jun 2021 in News
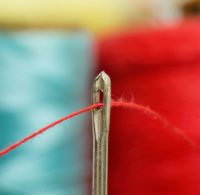
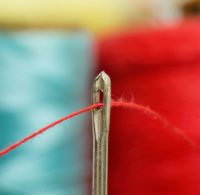
In this exclusive article, Aarti Bharadwaj, vice president, analytics, APAC at Essence, and judge of The Wires Global 2021, explains the value of brand analysts, and sets out what brands can do to optimise their data and analytics.
Data and analytics have, in recent years, become synonymous with terms like algorithmic optimisation and unsupervised machine learning on big datasets. More often than not, analytics offerings are spoken of as self-serve platforms where marketers are able to generate actionable insights from their data.
In marketing, data and analytics have many facets - from consumer-centric data, to behavioural data, to purchase behaviour data, and to media consumption or engagement data. Each of these datasets has its nuances, and is large and complex in its own right. Also, each dataset has a purpose and utility for a brand to articulate and operationalise its brand strategy.
Usage of data and analytics from marketers’ perspective
Let’s look at the usage of data and analytics from marketers’ perspective. As marketers articulate their brand strategy, they need to account for what has worked for them in the past, consider emerging trends, and predict how consumers will react in the future. In other words, each brand is unique in what they know and have measured, and in what they do not know and do not have experience in. Thus, each brand is unique in its learning agenda. As such, marketers need to be able to read and interpret each dataset, not just with the specific nuances of the research, but in the context of the brand, product lifecycle, and market dynamics as well.
Furthermore, it’s important to acknowledge that a large part of the learning agenda for a brand is informed by marketers’ intuition on the brand. Such wisdom and instinct are often informed, not by data, but by category dynamics or specific anecdotal evidence. Therefore, the role of a learning agenda for any brand is to consider this intuition and historical experience, and then confirm or negate the same in the data. This makes a large part of marketing analytics confirmatory in nature and not blue sky thinking.
Selecting the right tools and platforms is a big challenge
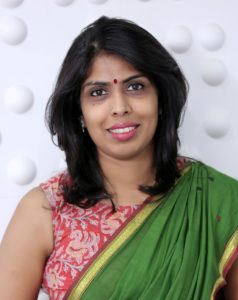
Aarti Bharadwaj, vice president, analytics, APAC at Essence
The recent proliferation of research and data sources on one hand, and analytics tools and platforms on the other, have exponentially increased the possibilities of data-driven value for businesses. At the same time, there are significant costs associated with acquiring the right data, and ensuring that it is properly archived and accessed by the right people. These involve significant investment in technology and engineering infrastructure and skills.
All this has exposed a need gap for marketers to shine a light on data that is of value to the brand and business, versus data that is just noise. The need is for brand analysts or consulting partners who can navigate the data ecosystem and make purposeful choices in terms of the types of data and granularity needed, as well as tailor the analysis to deliver speed and robustness from the insights derived. The role of the brand analyst is to maximise the value that marketers can extract from their data, making them akin to a chef - they need to understand the brand’s needs, and then choose the right ingredients and recipes that would cater to those requirements.
Five-point checklist to relevant data and analytics
The first step for brand analysts is to develop an understanding of the underlying business processes, and understand the known knowns and the known unknowns for the brand. Using this, they can develop a learning roadmap - a plan that prioritises the brand’s immediate analysis needs and learnings that need to be developed in the future.
The next step is to audit the data health of the organisation. The data assets of the brand, including data sources, metrics and infrastructure, should be studied in terms of the following factors:
- Purpose: It is necessary to establish whether the data sources and metrics captured for the brand are in line with its learning agenda. For instance, if the learning objective is to measure brand health in a highly dynamic category, marketers would need to know if their brand tracking is capturing the frequency of data required. The solution to staying relevant to category behaviours could lie in an agile online brand tracker.
- Coverage: It is crucial that the data is representative of all aspects of the business and its customers. For example, in a world where purchase behaviour is increasingly digital, it is imperative to have robust ecommerce data regarding the brand and category. This would serve as input into building out growth strategies which are future-facing and not biased by past behaviours. Another aspect to consider is whether the data covers all consumers, current and prospective, and their behaviours.
- Robustness: It is important to think about this aspect from the perspective of data sources, the database architecture, and the scalability of analysis. A robust data infrastructure would comprise a set of consistent data sources which maintain consistent metrics within a steady database setup. The analytics layer is built such that it is consistent with the underlying data and can be validated and replicated over time.
- Access: Data is most valuable when it is democratised. Therefore, one key aspect to consider is the end-user of the data and analysis. Additionally, there should be an audit of the number of users and the choice of infrastructure needs to be in line with the usage load.
- Security: Last but not least, database security needs to be aligned with legal regulations, and must comply with the policies and protocols of the business.
Once this data foundation has been laid, the possibilities with analytics range from simple visualisations to enable hypothesis-building, to complex and advanced data sciences. However, at each stage of analysis, the principles of purpose, coverage, and robustness should be considered to ensure that the recommendations are meaningful and will create value for the overall business.
Collaboration in a trusted ecosystem is key
It is straightforward to understand that no one individual would be able to comprehend and amalgamate a deep understanding of all business and consumer data. Successful data-driven strategies and businesses are co-created by diverse teams of trusted specialists who collaborate to bring together the required skills.
After all, while actual analysis of data is done by automated platforms and unstructured machine learning algorithms, it is still critical for brand analysts to orchestrate the data and analysis in a way that it is relevant, easily applicable, and provides valuable decision support to all of a brand’s stakeholders.
The Wires Global 2021 awards are now open for entry. Please visit our dedicated awards platform for further details and to enter.
AgencyAnalyticsBrandsDataMarketing
Follow ExchangeWire