Virtuous Advertising: Interview with Remi Lemonnier, Scibids
by Mathew Broughton on 12th Jul 2022 in News
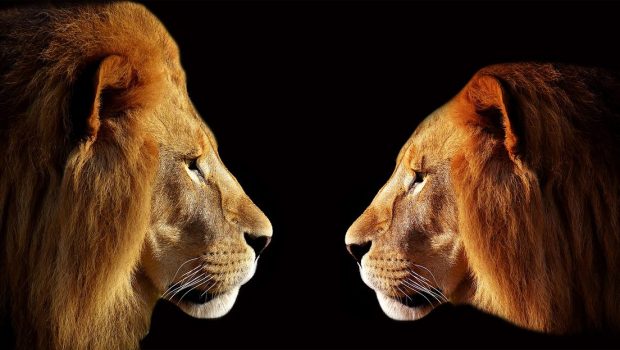
In association with Scibids.
Following a sneak peek of the results at ATS London 2022, ExchangeWire and Scibids have launched their latest Insights & Attitudes report: Solving UK Marketing Challenges with AI.
In this article, Rémi Lemonnier, co-founder and president at Scibids, expands on some of the key findings from the study and introduces how AI can be used to address these challenges in terms of data, the environment, and human capital, thus realising the newly-coined concept of virtuous advertising.
What key steps can the market take to fulfil their aspirations of virtuous advertising: respect for data; respect for environment & economic resources; and respect for mental health & human capital?
Clearly, these are very important aspirations, and are obviously larger than one solution, one silver bullet to solve everything. However, I see a common way to start tackling these challenges for media buyers, which is to simply make the most of existing resources, instead of just pulling more resources into a problem to solve it, as if these resources were infinite.
In terms of respect for data, it means we should be acknowledging that user data will become scarcer, and that using powerful AI to extract the most value from the remaining contextual data can be a solution which can have the same, or better, performance than before during the era of behavioural targeting.
Secondly, respect for the environment and reducing carbon emissions, this means acknowledging that "spray and pray" strategies cannot work anymore because we have to be conscious of the carbon footprint of an impression. Here, media buyers can use AI to identify and buy only the highest performing impressions. AI also comes into play to balance the potential trade-off between performance and reduction of carbon emissions.
Lastly, respect for health and human capital. To fulfil this aspiration may involve recognising that some repetitive tasks are better done by machines than humans. If you hire smart experts, it's not a good use of their time and intelligence to have them do a lot of hyper-repetitive tasks. Instead, it is better to use their expertise, which is becoming increasingly scarce, to add strategic value to a campaign, rather than manually whitelisting or blacklisting domains, for example.
Why is such a low proportion of the UK market using contextual signals present in log-level data, despite these being scalable and privacy-preserving? What more can be done to encourage the market to embrace these signals?
These signals have always been underused as far as I can tell, maybe the rationale behind that is that every media buyer can access them. Our position at Scibids is that it is not really the data you have that makes a difference, but how you use it. Log-level data can be accessed on any campaign, however, if you have it in your database but are not using it, or you are just using simple rules, obviously you don't get the performance out of it that you would get if you used sophisticated AI to analyse it. The market is always thinking a lot in terms of "What additional data can I have compared to my competitors?", without considering what additional capabilities are present to exploit that data.
How can AI be used to address widespread concerns among agency and brand professionals that there is a scarcity of expertise within the industry?
As managers, we have a duty to give our teams the right tools so that they can focus on where they add the most value. The Insights & Attitudes report reveals that UK market experts identify scarcity of expertise as the number one challenge for the industry. In terms of workload for instance, we all know that when we are recruiting, this means that the team is putting in longer hours prior to the position being filled. Clearly that that's something we have to fight to help them to keep a healthy balance between their personal and professional life.
The question is how can we equip our teams so that they are freed from repetitive tasks and also what insights we can give them, so that it elevates the quality of their workforce. AI is useful in not only improving the performance of a campaign, but also to improve the quality of insight on the campaign, meaning that an expert trader or campaign manager can understand exactly what's performing and what's not. Afterwards they can then go to the supply side and say, "Give me more of these deals, give me more of this potential context" so that everything works better and the human touch is used more effectively.
How can brands and agencies capitalise on their clear desire to reduce carbon emissions around their advertising efforts? How can technology solutions help drive down the large CPM carbon footprint of an impression?
Firstly, clearly one way to reduce carbon emissions is to just run fewer impressions by simply being more efficient in a campaign. I touched on this before, that AI can be used to select the best impressions, therefore it can be used to lower emissions by selecting fewer, but higher quality, impressions.
Apart from that, the very first step is to start measuring. Have a measurement partner that is going to be able to identify the contexts that are driving high carbon emissions. The next step is to look into the supply side and identify the publisher properties that have the highest carbon emissions. Clearly, a website which is slow and calls tonnes of different partners within its ads.txt file is going to have a much larger carbon footprint. As a media buyer, you can actually start integrating the carbon footprint of the supply side in the decisioning and in the bidding, and bid less when the carbon footprint of the supplier is high.
What steps can the industry take to adapt to upcoming changes in privacy policies, given the clear uncertainty in the market?
There are still uncertainties, we know what's going away, but all the initiatives to replace third-party cookies are still under construction and the technical specifications are not finished yet. However, what seems to be clear is that, in the long run, we have to consider that every data source that is not very clearly supporting a key use case of ad tech is going to disappear for privacy reasons. The question is, what is going to remain?
At Scibids, we think first-party data and a way for media buyers to perform attribution will remain, such as a method for media buyers to identify contexts performing well on the campaigns. This will come in the form of granular reports where, in every context, the number of impressions and positive events, with a certain level of aggregation and random noise added by the browser. This is still very good fuel for any learning algorithm to understand exactly the good from bad contexts for a campaign. Media buyers should ask themselves whether they are ready to work from these datasets which, while reduced, still convey the necessary information for a campaign to do well.
Artificial IntelligenceContextualEnvironmentalESGPrivacyUK
Follow ExchangeWire