AI Overload: Are We Spending Wisely on Artificial Intelligence?
by News
on 18th Dec 2024 in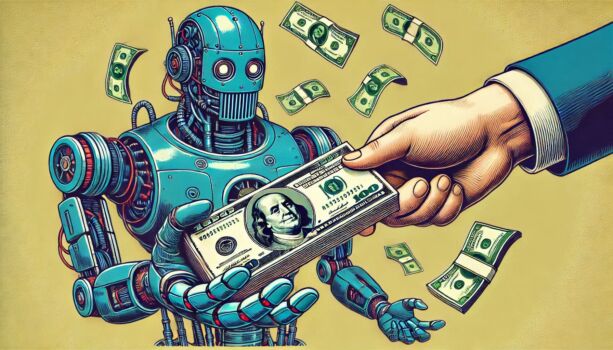
In this op-ed, James Heimers, SVP of analytics at RAPP, discusses the investment in artificial intelligence, and what the industry can do to make AI work.
The explosive growth in AI investment has been both lauded as transformative and criticised as overhyped. The promise of AI to unlock new levels of efficiency, personalisation, and insight has driven massive financial commitments. But there is a growing discourse on whether this surge of investment has truly delivered on its promises or if it has resulted in a "tech overhang", where the anticipated benefits lag behind the resources deployed.
The unfulfilled promise of big data
A recent Goldman Sachs report entitled Gen AI: Too much spend, too little benefit? highlights a significant concern – tech giants and beyond are set to spend over USD$1tn (£8bn) on AI capital expenditure in the coming years, with little to show for it so far. MIT’s Daron Acemoglu and Goldman Sachs’ Jim Covello are sceptical about AI's ability to generate significant economic upside or solve complex problems that justify such high costs. This scepticism mirrors the broader issue of big data’s unfulfilled promise. The vast amounts of data collected have not consistently translated into actionable insights or substantial business benefits, leading to the "tech overhang".
Mere collection of data is insufficient. There needs to be a deeper causal understanding of marketing effectiveness. By integrating diverse data sources, we can better understand the drivers behind customer behaviour and optimise marketing strategies accordingly.
The human element and the empathy gap
While some experts remain optimistic about AI's long-term benefits, others point to current limitations including the lack of a "killer application" that justifies the investment. This aligns with the concept of the "empathy gap" – where businesses' promises far exceed their deliverables, leading to customer disillusionment. The scope-severity paradox highlight our diminishing sensitivity to outcomes as numbers grow. These concepts are crucial in understanding why large datasets might not always lead to better human connections or business outcomes.
Contextual clarity and the right data
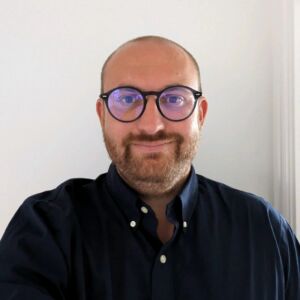
The Goldman Sachs report suggests that the issue is not AI itself but rather how it is used. This is echoed in the importance of contextual clarity – understanding that customer behaviour is influenced by various external and macro factors beyond what is visible in first-party data. So first-party data must be enriched with other datasets across organisations, as well as third-party customer, competitor, and macro data to provide a more comprehensive view of customer behaviour. This approach will help businesses predict impacts and make informed decisions, ultimately leading to better marketing strategies and improved ROI.
Building a strong AI foundation for sustainable growth
There are critical components needed to create a strong AI foundation that supports sustainable growth. This involves not just technology, but a strategic and cultural shift within organisations. Here are some key takeaways:
- Strategic approach: Avoid getting enamoured by the latest shiny objects in AI. Instead, focus on integrating AI strategically and sustainably into your business processes.
- Connected data, decisioning, and content: AI can supercharge creative output and drive growth when data, decision-making, and content creation are seamlessly connected.
- Governance and ethics: Establish robust governance and ethical guidelines to ensure AI is used responsibly, mitigating risks such as bias, data privacy concerns, and intellectual property issues.
- Cultural buy-in: Foster a culture that embraces experimentation and is willing to fail and learn quickly. This agile approach can help businesses adapt to the fast-evolving AI landscape.
From data to empathy: creative testing and human insight
To address the gap between AI's potential and its current impact, businesses should refocus on the human element and have a clear use case to create value for the end-user in a business or for customers.
One example is using AI for testing creative, to ensure the most impactful work is produced. Creative testing involves a structured approach to ensure marketing campaigns resonate with their intended audiences before full-scale launch. This can be done through a combination of first-party data and using robust and representative consumer panels of intended target audiences.
By profiling panels, their geo-demographic, socio-economic, and personality traits can be better understood. Which means as new content is generated, businesses can begin pre-launch testing of campaigns, creative, and messaging variants with panels to identify which variants are most likely to deliver the highest performance. This includes evaluating ease of understanding, relatability, likeability, interest, likelihood to engage, ownability, differentiation, and meaningfulness.
From this point AI can analyse and optimise to understand the performance through a combination of content intelligence, neuroscience, and eye tracking. Which means going forwards AI-generated scores can help identify the best-performing content variants, optimise pre-launch, and make more precise in-flight adjustments during the campaign.
By starting with pre-tested content and continuously optimising based on human feedback and AI insights, we can launch campaigns that are more precise, perform better, and achieve greater audience conversion.
Balancing data and human insight
While organisations are heavily investing in AI, the return on this investment will only be realised when businesses learn to balance data with human insight. There is certainly a need for a more nuanced approach to investing in AI, one that prioritises human connection and contextual understanding as much as advancing technology. By focusing on contextual clarity, empathy, and the right use cases, businesses can transform AI from a buzzword into a tool that genuinely improves business success. The key is not more data, but smarter, more empathetic use of the data we have.
AIContextualDataFirst-Party Data
Follow ExchangeWire